Applications and limitations of linear response functions in models of neural circuits
All functions of the brain are generated by neural circuits with complex nonlinear interactions and feedback loops. In the past decades, many of them were successfully described by less complex approaches based on linear response functions. Tim Herfurth and Tatjana Tchumatchenko from the Theory of Neural Dynamics Group (Max Planck Institute for Brain Research) now dive into the wide variety of applications and the limitations of these models and discuss why alternatives are needed and what they may be.
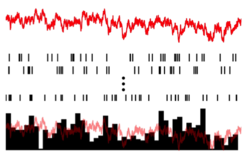
Linear response functions were originally developed to understand phenomena in physics and have become an important tool in neuroscience. They lead to deeper insight into diverse areas of neuroscience such as rate dynamics, synchrony, global oscillations, and information theory. Herfurth: "Linear response functions have been applied to successfully predict the firing activity for a given sensory stimulus, e.g. the response of a retina to a light stimulus. These functions were also useful in determining when and how neurons will synchronize or how much information they transmit".
However, the linear response concept is now facing its limitation because it was originally developed for systems which fulfill certain stationarity criteria or which are subject to weak stimuli. For many processes in the brain, these do not apply and functions such as classification, adaptation or stimulus-dependent normalization require additional nonlinear contributions. Tchumatchenko: "The linear response theory makes predictions only about firing rates but not about changes in spike timing coordination, which is considered a fundamental unit of neural computation. One way to overcome these limitations is to add non-linearity and feedback loops".